Spatial Relationality: Bacon Tables
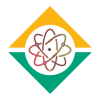
December 19, 2024 2 minutes • 385 words
Table of contents
Chapter 9 explained that Einstein’s system for judging space is to use the metric tensor. These are small arrows that go from Point A to Point B.
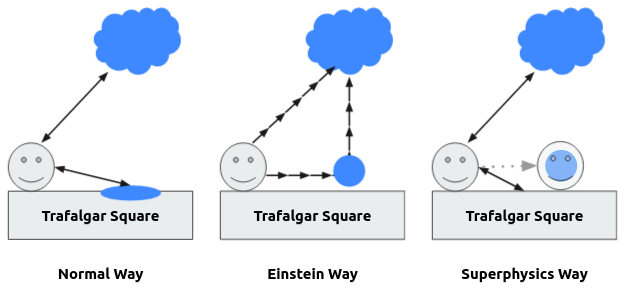
But to Material Superphysics, the proper and natural way to judge the space of a cloud on Trafalgar square is to know the nature of both:
- the cloud, and
- Trafalgar square
In this way, the proper location is determined by the relative differences of those two natures.
A blind observer can know the cloud by the thunder it creates and how it compares to other sounds from Trafalgar square which he learns by experience. In this way, this system can work even without light.
The Aethereal, Non-Radiant, Experiential Way
Assuming Trafalgar square has four squares A1, A2, B1, B2
. The person has existed in all squares and has heard thunder above each one, as well as above all the other squares. This leads to 16 total experiences.
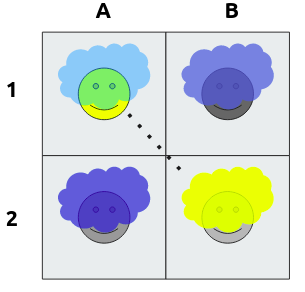
In this way, the person in A1
who hears a thunder in B2
will know its location by extracting the relation of the cloud and the area from his memory.
- This relation is spatial relationality.
This method does away with the use of light as the measuring rod, and so it can throw away c
and lambda
while still being within the nature of the universe.
Instead of c, it uses the relation of the person in two states (A1 and B2) with the state of the cloud in B2.
Notice how this system is similar to quantum mechanics which assigns a probability to each location through a ‘path integral’ formulation.
The Francis Bacon Tables
The list of experiences of the blind man in our example allowed him to know which square the lightning came from.
This is the same technique that spatial relationality will use in order to simplify the judgement of space.
These experiences form a table which plots the relevant data needed to judge the space or movement in question.
Nowadays, this is known as the training data used by machine learning models.
- The data must be cleaned to match the model.
This means that our system will answer questions about space and movement based on training data instead of simple inputs like mass, velocity, distance, etc.